Our lab has several published and ongoing efforts to discover and validate biomarkers in T1D. Biomarkers are needed to predict the rate of progression from autoantibody positivity to clinical diagnosis or the rate of loss of insulin secretion after diagnosis, as well as to predict responders to therapy. Many investigators are working hard to identify new potential biomarkers, but moving them toward clinical utility has been very challenging.
We are interested in assessing the reproducibility of proposed biomarkers. This has been done internally at our lab, by sharing samples from our biorepository with others, and in workshops comparing assay results in multiple participating laboratories. Technical validation work like this is critical to ensure that the biomarkers identified now can be measured with enough reliability to eventually be useful in patient settings.
We led a study to test 12 different assays in 10 different laboratories around the world, and identified those assays and markers that met our requirements for technical performance. We then created a novel analysis tool in collaboration with those 10 labs, and developed a model that could predict rate of decline in insulin secretion after diagnosis.
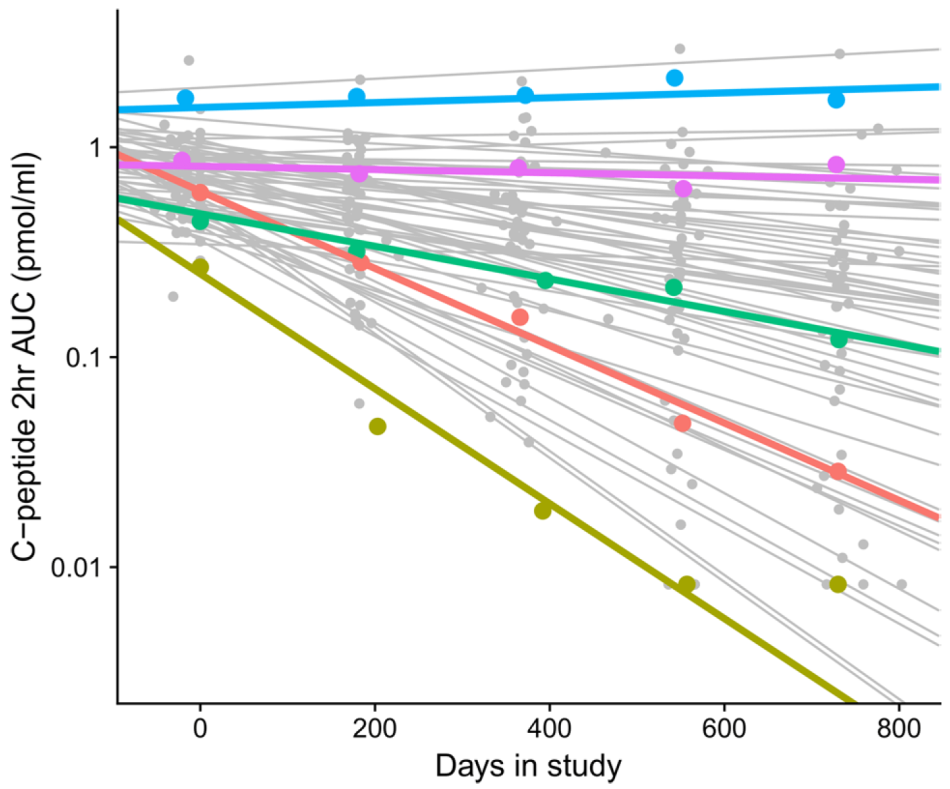
More recently, we have been developing novel assay methods and analytical approaches to advance cytometric assays. One example of this is the addition of person-specific markers to flow cytometry/CyTOF assays that allows for the detection of sample swaps in longitudinal data. At the moment this allows us to use cleaner data to understand mechanisms of disease – and in the long term it could be useful to detect sample swaps in clinical cytometry assays.
We also have ongoing work to reconsider the way data are analyzed in antigen-specific T cell studies in diabetes. These cells are measured in laboratories all over the world; they are incredibly important for our understanding of the biology of T1D and also represent potential outcome measures for clinical trials of antigen-specific therapies. However, longitudinal data have seldom been published from these assays in the context of trials, in part because the cells are highly numerically variable. We’ve been working on an approach to reconsider how these data can be analyzed to help move these cells – regardless of who they’re measured by – forward toward clinical utility.
Featured Publications
-
Dec 2019
A composite immune signature parallels disease progression across T1D subjects.
JCI InsightSpeake C, Skinner SO, Berel D, Whalen E, Dufort MJ, Young WC, Odegard JM, Pesenacker AM, Gorus FK, James EA, Levings MK, Linsley PS, Akirav EM, Pugliese A, Hessner MJ, Nepom GT, Gottardo R, Long SA -
Mar 2020
Circulating Unmethylated Insulin DNA As a Biomarker of Human Beta Cell Death: A Multi-laboratory Assay Comparison.
J Clin Endocrinol MetabSpeake C, Ylescupidez A, Neiman D, Shemer R, Glaser B, Tersey SA, Usmani-Brown S, Clark P, Wilhelm JJ, Bellin MD, Herold KC, Mirmira RG, Dor Y, Evans-Molina C -
Apr 2022
A simple strategy for sample annotation error detection in cytometry datasets.
Cytometry ASmithmyer ME, Wiedeman AE, Skibinski DAG, Savage AK, Acosta-Vega C, Scheiding S, Gersuk VH, O'Rourke C, Long SA, Buckner JH, Speake C
Additional Research Projects
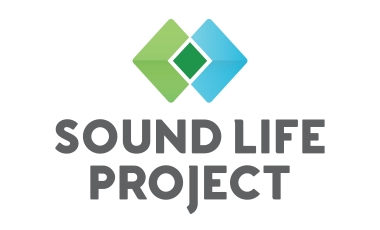
The Sound Life Project
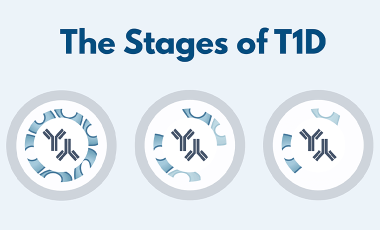